This is the second release of my newsletter. I’ve collected some papers, articles and vulnerabilities that were released in last two weeks, this time the resources are categorized into following categories: LLM Security, AI Safety, AI Security. If you are not a mail subscriber yet, feel invited to subscribe: https://hackstery.com/newsletter/.
Order of the resources is random.
Any feedback on this newsletter is welcome – you can mail me or post a comment in this article.
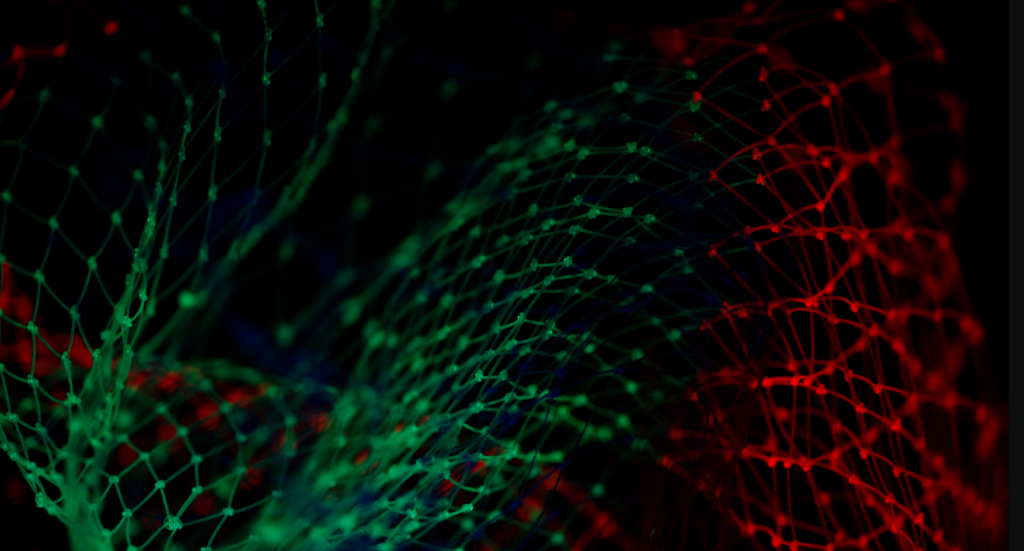
LLM Security
Image to prompt injection in Google Bard
“Embrace The Red” blog on hacking Google Bard using crafted images with prompt injection payload.
Link: https://embracethered.com/blog/posts/2023/google-bard-image-to-prompt-injection/
Paper: Challenges and Applications of Large Language Models
Comprehensive article on LLM challenges and applications, with a lot of useful resources on prompting, hallucinations etc.
Link: https://arxiv.org/abs/2307.10169
Remote Code Execution in MathGPT
Post about how Seungyun Baek hacked MathGPT.
Link: https://www.l0z1k.com/hacking-mathgpt/
AVID ML (AI Vulnerability Database) Integration with Garak
Garak is a LLM vulnerability scanner created by Leon Derczynski. According to the description, garak checks if an LLM will fail in a way we don’t necessarily want. garak probes for hallucination, data leakage, prompt injection, misinformation, toxicity generation, jailbreaks, and many other weaknesses. AvidML supports integration with Garak for quickly converting the vulnerabilities garak finds into informative, evidence-based reports.
Link: https://avidml.org/blog/garak-integration/
Limitations of LLM censorship and Mosaic Prompt attack
Although censorship brings negative associations, in terms of LLMs it can be used to prevent LLM from creating malicious content, such as ransomware code. In this paper authors demonstrate attack method called Mosaic Prompt, which is basically splitting malicious prompts into sets of non-malicious prompts.
Link: https://www.cl.cam.ac.uk/~is410/Papers/llm_censorship.pdf
Security, Privacy and Ethical concerns of ChatGPT
Yet another paper on ChatGPT 🙂
Link: https://arxiv.org/pdf/2307.14192.pdf
(Ab)using Images and Sounds for Indirect Instruction Injection in Multi-Modal LLMs
Using images and sounds for Indirect Prompt Injections. In this notebook you can take a look at the code used for generating images with injection: https://github.com/ebagdasa/multimodal_injection/blob/main/run_image_injection.ipynb
(I’ll be honest, it looks like magic)
Link: https://arxiv.org/abs/2307.10490
Universal and Transferable Adversarial Attacks on Aligned Language Models
Paper on creating transferable adversarial prompts, able to induce objectionable content in the public interfaces to ChatGPT, Bard, and Claude, as well as open source LLMs such as LLaMA-2-Chat, Pythia, Falcon, and others. This paper was supported by DARPA and the Air Force Research Laboratory.
Link: https://llm-attacks.org/zou2023universal.pdf + repo: https://github.com/llm-attacks/llm-attacks/tree/main/experiments
OWASP Top10 for LLM v1.0
OWASP released version 1.0 of Top10 for LLMs! You can also check my post on that list here.
Survey on extracting training data from pre-trained language models
Survey based on more than 100 key papers in fields such as natural language processing and security, exploring and systemizing attacks and protection methods.
Link: https://aclanthology.org/2023.trustnlp-1.23/
Wired on LLM security
This article features OWASP Top10 for LLM and plugins security concerns.
Link: https://www.wired.com/story/chatgpt-plugins-security-privacy-risk/
AI Safety
Ensuring Safe, Secure, and Trustworthy AI
Amazon, Anthropic, Google, Inflection, Meta, Microsoft and OpenAI have agreed to self-regulate their AI-based solutions. In these voluntary commitments, the companies pledge to ensure safety, security and trust in artificial intelligence.
Red teaming AI for bio-safety
Anthropic’s post on red teaming AI for biosafety and evaluating models capabilities i.e. for ability to output harmful biological information, such as designing and acquiring biological weapons.
Link: https://www.anthropic.com/index/frontier-threats-red-teaming-for-ai-safety
AI Security
AI Vulnerability Database releases Python library
According to documentation: “It empowers engineers and developers to build pipelines to export outcomes of tests in their ML pipelines as AVID reports, build an in-house vulnerability database, integrate existing sources of vulnerabilities into AVID-style reports, and much more!”
Link: https://twitter.com/AvidMldb/status/1683883556064616448
Mandiant – Securing AI pipeline
The article from Mandiant on securing the AI pipeline. Contains GAIA (Good AI Assessment) Top 10, a list of common attacks and weaknesses in the AI pipeline.
Link: https://www.mandiant.com/resources/blog/securing-ai-pipeline
Google paper on AI red teaming
Citing the summary of this document:
“In this paper, we dive deeper into SAIF to explore one critical capability that we deploy to support the SAIF framework: red teaming. This includes three important areas:
1. What red teaming is and why it is important
2. What types of attacks red teams simulate
3. Lessons we have learned that we can share with others”
Link: https://services.google.com/fh/files/blogs/google_ai_red_team_digital_final.pdf
Probably Approximately Correct (PAC) Privacy
MIT researchers have developed a technique to protect sensitive data encoded within machine learning models. By adding noise or randomness to the model, the researchers aim to make it more difficult for malicious agents to extract the original data. However, this perturbation reduces the model’s accuracy, so the researchers have created a framework called Probably Approximately Correct (PAC) Privacy. This framework automatically determines the minimal amount of noise needed to protect the data, without requiring knowledge of the model’s inner workings or training process.
Link: https://news.mit.edu/2023/new-way-look-data-privacy-0714